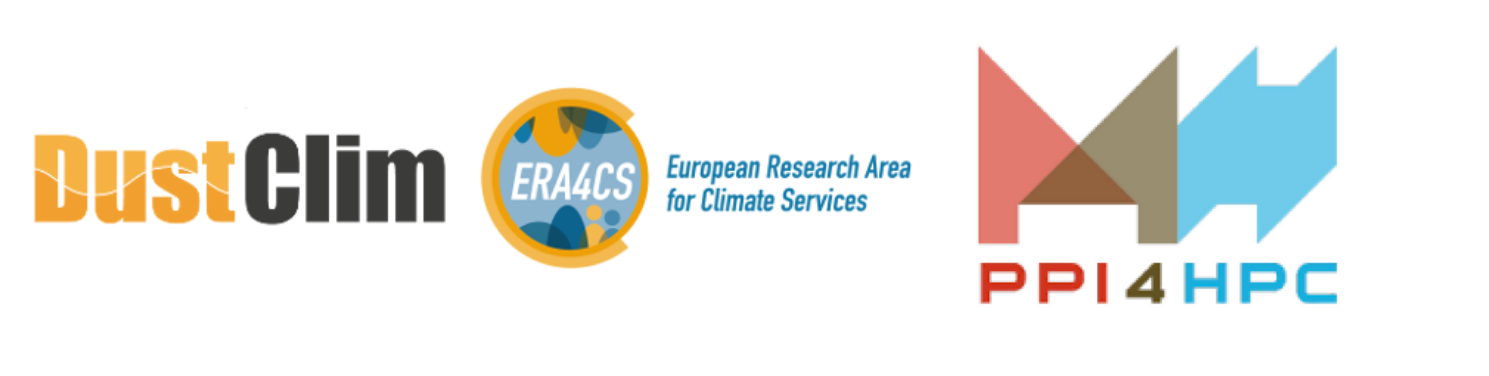
Written by Kim Serradell Maronda and Francesca Macchia from BSC
In alignment with the World Meteorological Organization (WMO) mission, the “Dust Storms Assessment for the development of user-oriented Climate Services in Northern Africa, Middle East and Europe“ project (DustClim) made a major step forward in the way sand and dust storms (SDS) affect society, using the storage infrastructure acquired through the public procurement of the PPI4HPC project.
Taking benefit of the mass storage services provided by the PPI4HPC system at the Barcelona Supercomputing Center (BSC), the DustClim project developed dust-related services for the air quality, aviation and solar energy sectors and produced and delivered an advanced and thoroughly evaluated decadal high-resolution dust reanalysis for Northern Africa, Middle East and Europe.
SDS are an important threat to health, property, equipment, and the economy in many countries, whereas the scales of the threat can be considerable. In many cases, the risks include loss of lives, substantial disruptions of activities and operations, and economic losses. Reacting to the concerns about SDS by its most affected member states, the WMO promoted the delivery of timely and quality SDS forecasts, observations, information and knowledge to users through an international partnership of research and operational communities.
Understanding desert dust impacts requires fundamental and cross-disciplinary knowledge underpinned by state-of-the-art scientific research, the availability of reliable information on past trends and current conditions. A major obstacle to reconstructing comprehensive dust information of the past is the scarcity of historical and routine in-situ dust observations, particularly in the countries most affected by desert dust. Model simulations can be used to “fill in the blanks” and overcome the sparse coverage, low temporal resolution, and partial information provided by measurements.
The novelties of the DustClim reanalysis include its unprecedented high-resolution, the assimilation of satellite products over dust source regions with specific dust observational constraints, and a thorough evaluation using a wide variety of observations and data from experimental campaigns. It was produced using the Multiscale Online Non-hydrostatic AtmospheRe CHemistry model (MONARCH) coupled to a Local Ensemble Transform Kalman Filter (LETKF) data assimilation scheme [1] developed at the Barcelona Supercomputing Center (BSC), and assimilating satellite observations of dust optical depth from MODIS Deep Blue retrievals at 10 km resolution [2]. The simulations were performed on 1-day chunks, composed of a forecast step (12 ensemble member of MONARCH forward simulations), a state estimation step (LETKF data assimilation), and two post-processing steps needed to compress the original model output and to calculate ensemble statistics. The submission of the jobs was automatically handled by Autosubmit, a python-based workflow manager that allows creating, managing, and monitoring experiments remotely [3]. Autosubmit wrapped all the steps of 30 consecutive days in a single job submission requiring 10320 cores for ~24 hours. Different experiments were created, each of them performing a 2-month spinup and 1-year reanalysis. The web app Autosubmit GUI was used to easily monitor the experiments [4].
A 10-year data set of desert dust at a horizontal resolution of 0.1° was produced, covering the period 2007-2016. Beside 3D fields of dust mass concentration, the reanalysis data set includes dust extinction and deposition variables, plus other meteorological and radiation variables. More than 500TB of daily 3-hourly output files were stored and evaluated with various observations from ground-based and satellite observations. Also, a set of ensemble statistics are calculated and archived for each output variable. Statistics of analysis departures from assimilated observations prove the consistency of the data assimilation system showing that the analysis is closer to the observations than the first-guess. Temporal means of analysis increments show that the assimilation led to an overall reduction of dust with a pattern of systematic corrections that vary with the seasons, and can be linked primarily to misrepresentation of source strength. Independent evaluation of the analysis with AERONET observations indicates that the reanalysis data set is highly accurate, and provides therefore a reliable historical record of atmospheric desert dust concentrations in the recent decade.
Using a high-performance storage system, acquired by the PPI4HPC project, has been a critical factor in accomplishing this project. From the generation of the files by the model to the post-process stage of extracting final products, we need an efficient filesystem with enough throughput to process such a massive volume of data. And finally, when the whole experiment is over, the produced data can be stored in the archive system for further analysis.
The reanalysis data set can be used in support of climate services and monitoring. It can also aid research and the development of mitigation strategy. More specifically, the design of the reanalysis output fields has been tailored to the needs of specific services in the domain of three socio-economic sectors particularly affected by mineral dust such as health, energy production and transport. Also, the work done in the framework of DustClim is being referenced in UN committees and initiatives that search to assess the desert dust impacts and its associated risk.
References:
[1] Di Tomaso, E., Schutgens, N. A. J., Jorba, O., and Pérez García-Pando, C.: Assimilation of MODIS Dark Target and Deep Blue observations in the dust aerosol component of NMMB-MONARCH version 1.0, Geosci. Model Dev., 10, 1107–1129, https://doi.org/10.5194/gmd-10-1107-2017, 2017.
[2] Pu, B. and Ginoux, P. : The impact of the Pacific Decadal Oscillation on springtime dust activity in Syria. Atmos Chem Phys 16, 13431–13448, doi: 10.5194/acp-16-13431-2016, 2016.
[3] D. Manubens-Gil, J. Vegas-Regidor, C. Prodhomme, O. Mula-Valls and F. J. Doblas-Reyes, "Seamless management of ensemble climate prediction experiments on HPC platforms," 2016 International Conference on High Performance Computing & Simulation (HPCS), Innsbruck, 2016, pp. 895-900. doi: 10.1109/HPCSim.2016.7568429.
[4] Uruchi et al., (2021). Autosubmit GUI: A Javascript-based Graphical User Interface to Monitor Experiments Workflow Execution. Journal of Open Source Software, 6(59), 3049, https://doi.org/10.21105/joss.03049.